Hey there, tech enthusiasts and curious minds! Remember when we thought training AI was as cheap as your morning latte? Well, grab your wallet, because the latest numbers are going to make your head spin!
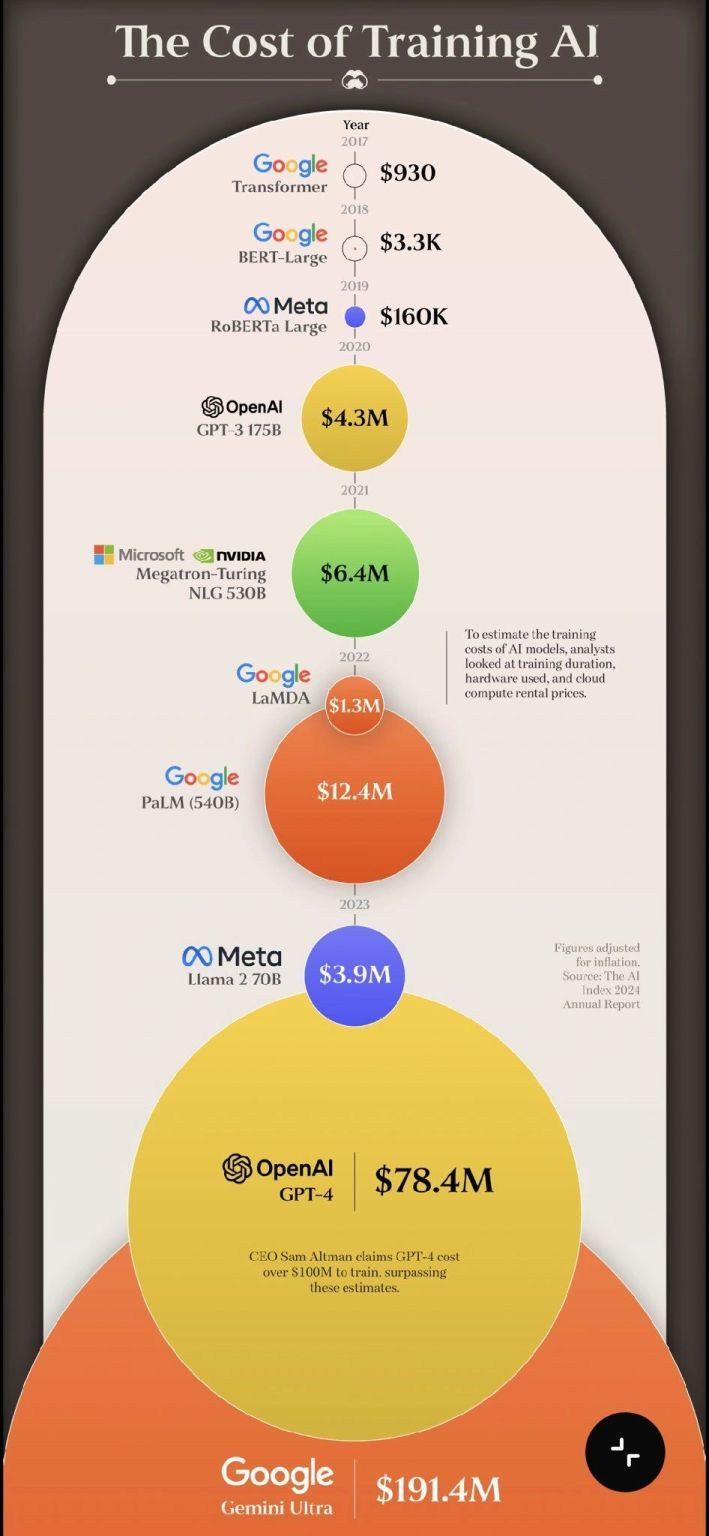
Image source : https://aiindex.stanford.edu/report/
The Price Tag of Digital Brains: From Ouch to OUCH!
The Stanford 2024 AI Index Report just dropped some mind-blowing figures:
- Original Transformer Model: $930
- GPT-3: $4.3 million
- GPT-4: $78.4 million
- Gemini Ultra: $191.4 million
And hold onto your hats, folks – there are whispers that GPT-5 could cost over $1 BILLION! 🤯
The Secret Ingredients of an AI Genius (and Why They Cost a Fortune)
1. Data: The All-You-Can-Eat Buffet of Information
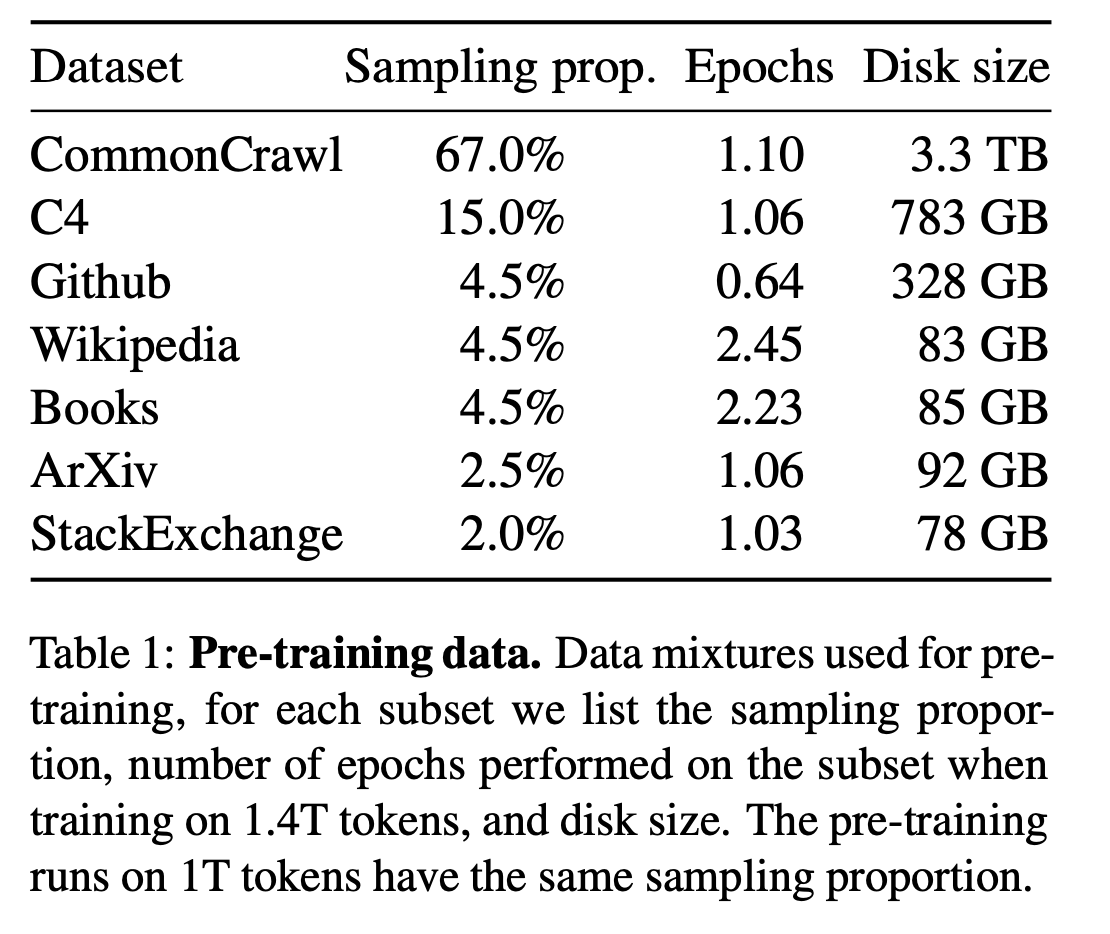
Imagine trying to read every book, website, and tweet ever written – that’s basically what we’re asking these AIs to do. We’re talking terabytes upon terabytes of data that need to be:
- Collected: Scouring the internet for every bit of useful info
- Cleaned: Getting rid of the junk (and trust me, there’s a lot of it)
- Organized: Putting everything in neat little digital boxes
This isn’t just a quick Google search – it’s more like organizing the world’s biggest library… blindfolded… underwater.
2. The Brainpower Behind the Artificial Brains
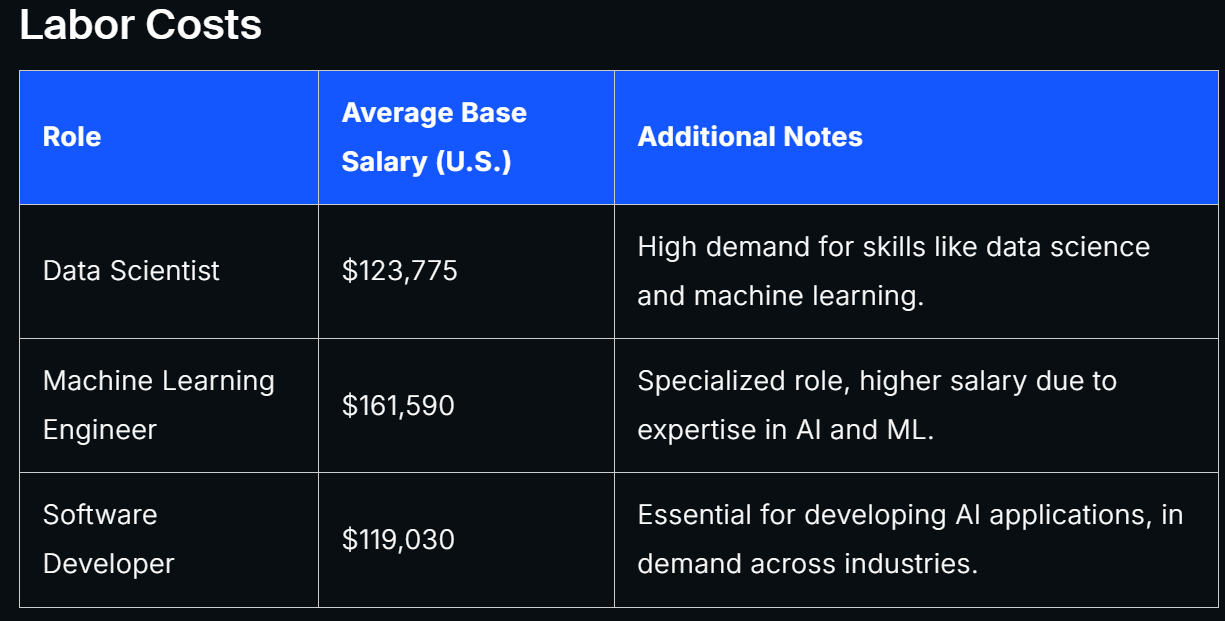
Building an AI isn’t a job for your average computer whiz. We’re talking about the Einsteins of the coding world. They don’t come cheap:
- Some top AI researchers are raking in up to $10 million a year!
- You need a whole team of these geniuses: machine learning wizards, data science ninjas, and language experts who speak “computer.”
3. Supercomputers: The Hungry, Hungry Hippos of the Tech World
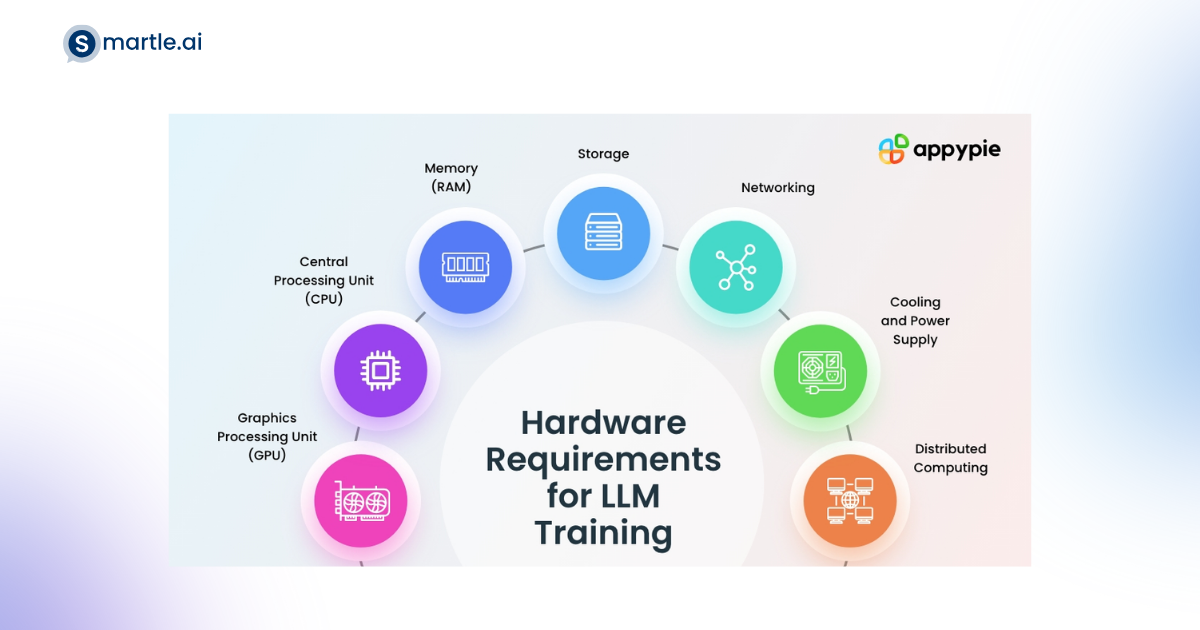
Training an AI like GPT-4 isn’t just intense – it’s like running a small city’s worth of computers at full blast for months:
- We’re talking thousands of high-powered GPUs (those things that make your video games look pretty)
- They run 24/7, gobbling up electricity like there’s no tomorrow
- The electric bill alone could probably power a small country for a year
4. Size Matters (in AI Land)
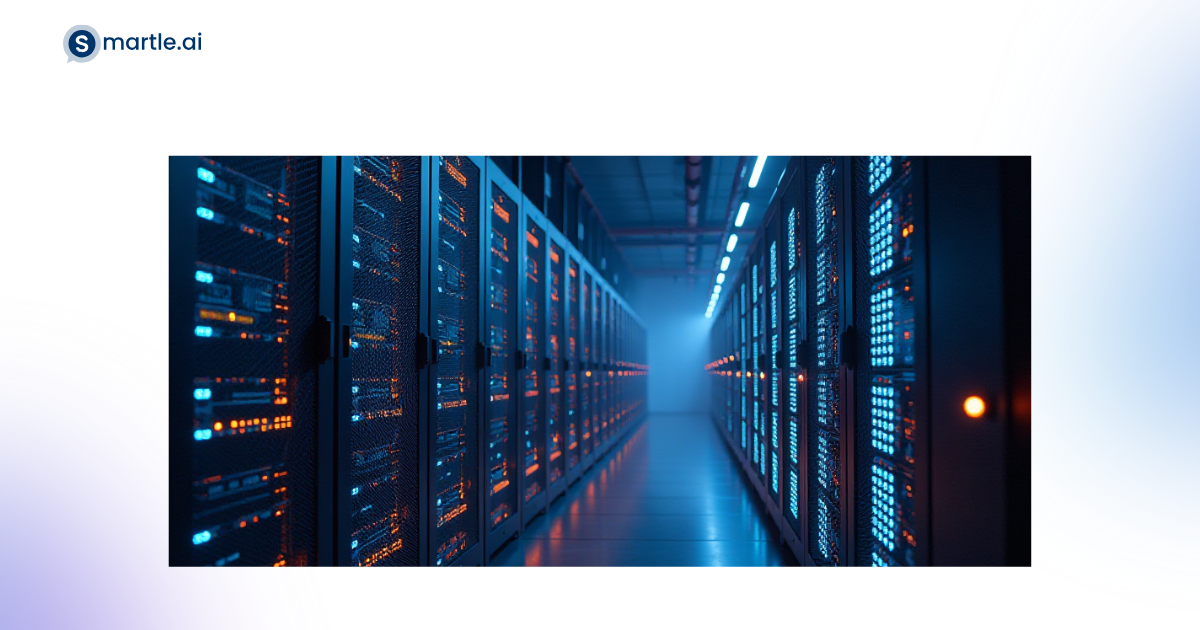
The larger the AI model, the more impressive its capabilities, but this also means a steep increase in costs. Bigger models have more parameters—essentially, the “knobs” and “dials” that determine how the AI thinks. More parameters equal more data, more computing power, and more money. It’s a scaling issue:
- More Data: With every leap in size, models require exponentially more data. This isn’t just about scraping the web; it’s about accessing proprietary datasets that can cost millions.
- More Parameters: GPT-3 has 175 billion parameters. Compare that to smaller, more efficient models like those used in specialized applications, and you realize the bulk of these massive models.
- More Computation: Larger models require more time to train, more GPUs, and more electricity. Imagine an army of GPUs working around the clock, each gulping power like an electric car constantly revving its engine. The training costs reflect this, ballooning into the millions for the top-tier models.
But Wait, There’s More (Cost)!
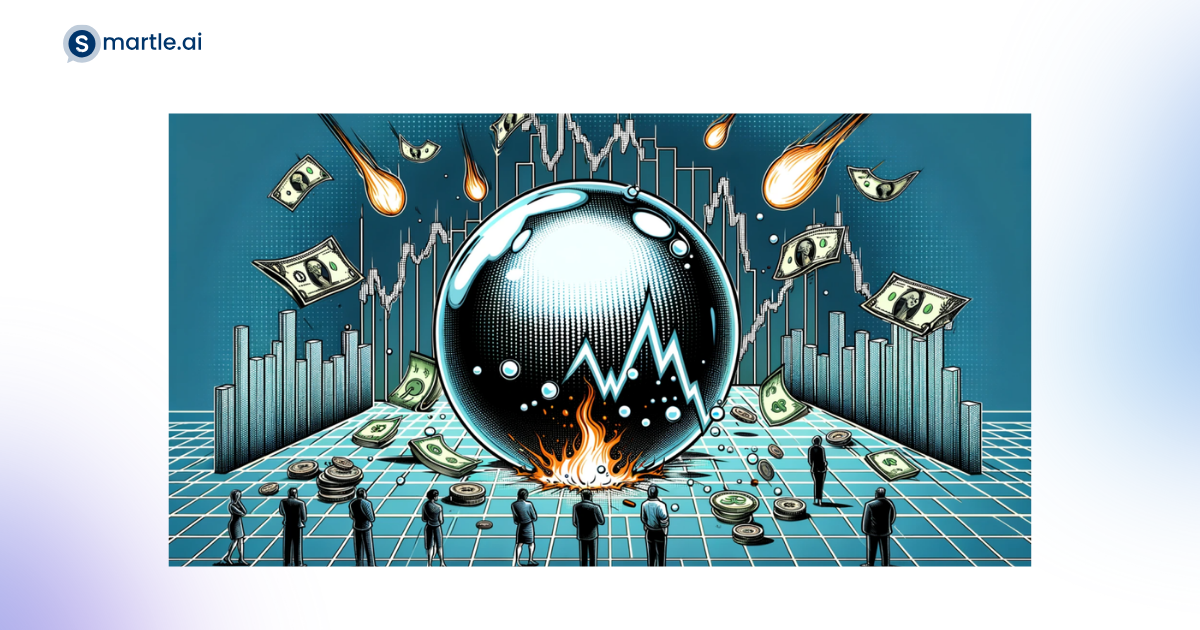
Think that’s the end of it? Let’s dive deeper into this money pit:
- Daily Burn Rate: OpenAI is reportedly spending up to $700,000 PER DAY just on server costs.
- Energy Hunger: The future of AI might need 30 TIMES of our current grid capacity. We’re talking about “buy a nuclear power plant” levels of energy demand!
- Talent Doesn’t Come Cheap: Top AI researchers are commanding salaries up to $10 million a year.
The Billion-Dollar Question: Is It Worth It?
As these costs keep skyrocketing, we’ve got to ask: Are we getting our money’s worth, or are we just feeding an insatiable digital beast?
The Optimists Say:
“This is just the cost of innovation!”
“Once we crack true AI, it’ll be worth every penny!”
“Quantum computing will make this all cheaper… eventually.”
The Skeptics Counter:
“Current LLMs can’t unlearn or correct themselves. They’re stuck with their training data.”
“We’re just introducing new flaws at increased costs.”
“True AI needs to adapt to changing conditions, not just regurgitate fixed datasets.”
The Dot-Com Bubble 2.0?
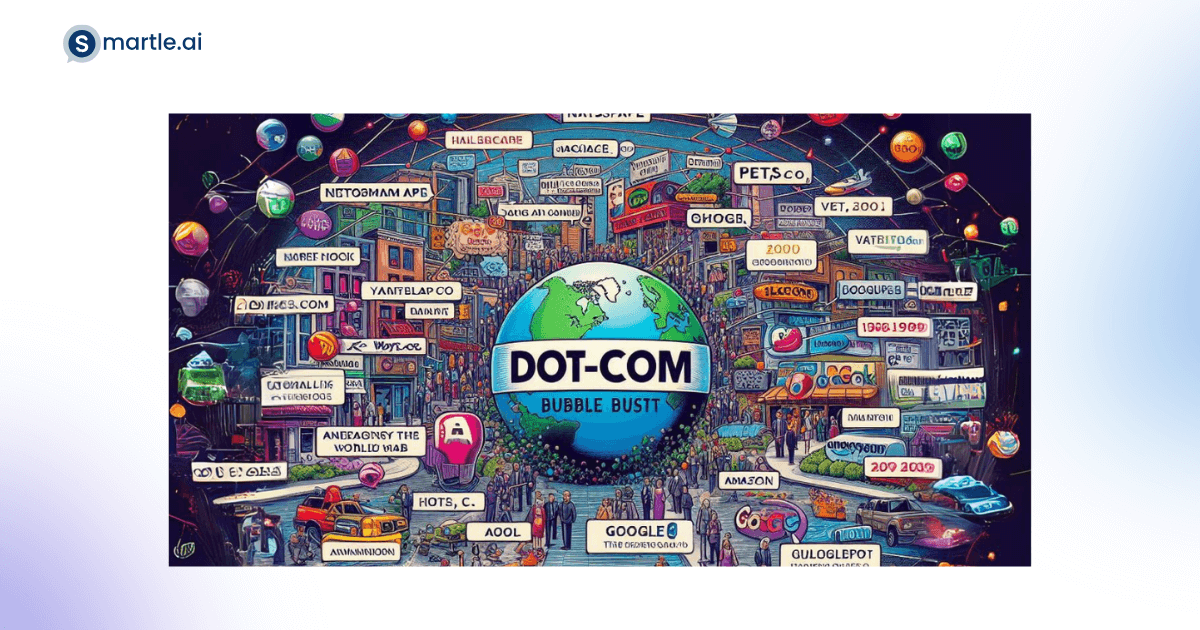
Some are drawing parallels to the dot-com era. Remember the cycle?
- Overhype and overpromise
- Burn money like there’s no tomorrow
- Chase users and growth at all costs
- Investors get antsy and demand profits
- 90% of startups realize their business model is as stable as a house of cards
Sound familiar? 🤔
Strategy: Companies can avoid the AI bubble by focusing on sustainable growth and practical applications. Leveraging existing AI models for specific, real-world tasks provides immediate value, proving that AI can enhance business operations rather than becoming a financial sinkhole. This strategy also helps in building more resilient business models that are not solely dependent on continuous investment in unproven AI technologies.
So, What’s Next?
- Efficiency is Key: As one commenter pointed out, hardware and software improvements could make training 2-4 times cheaper in just a couple of years. Maybe we need to slow down and let the tech catch up. Way Forward: Embrace ongoing advancements in hardware and optimization techniques to reduce training costs. By focusing on efficiency, businesses can take advantage of more affordable AI capabilities as technology evolves.
- Open Source to the Rescue?: While OpenAI ironically became very closed, open-source models like Meta’s LLaMA 2 are gaining traction. Could this democratize AI development? Approach: Adopting open-source AI models can drastically reduce costs and allow businesses to customize and scale AI solutions without proprietary restrictions. This fosters innovation and enables a wider range of companies to participate in the AI revolution.
- Focus on the Data: As one wise soul said, “It is the data itself that ultimately matters.” Maybe instead of bigger models, we need smarter data strategies? Way Forward: Prioritize data quality over quantity. Implement smart data strategies that focus on collecting relevant, high-quality data to improve AI performance. This approach ensures that AI models remain efficient and effective, without the need for excessive data collection and storage costs.
- The Human Touch: Let’s not forget – human brains are still the champs at making trade-offs and decisions. Maybe the future isn’t just bigger AI, but better human-AI collaboration. Strategy: Integrate human expertise with AI capabilities to create powerful hybrid systems. By leveraging human intuition and decision-making alongside AI’s computational power, businesses can achieve more nuanced and effective outcomes, especially in complex scenarios that require ethical considerations and empathy.
Smart AI Investments: Unlocking the Power of Billion-Dollar AI Models for Your Business
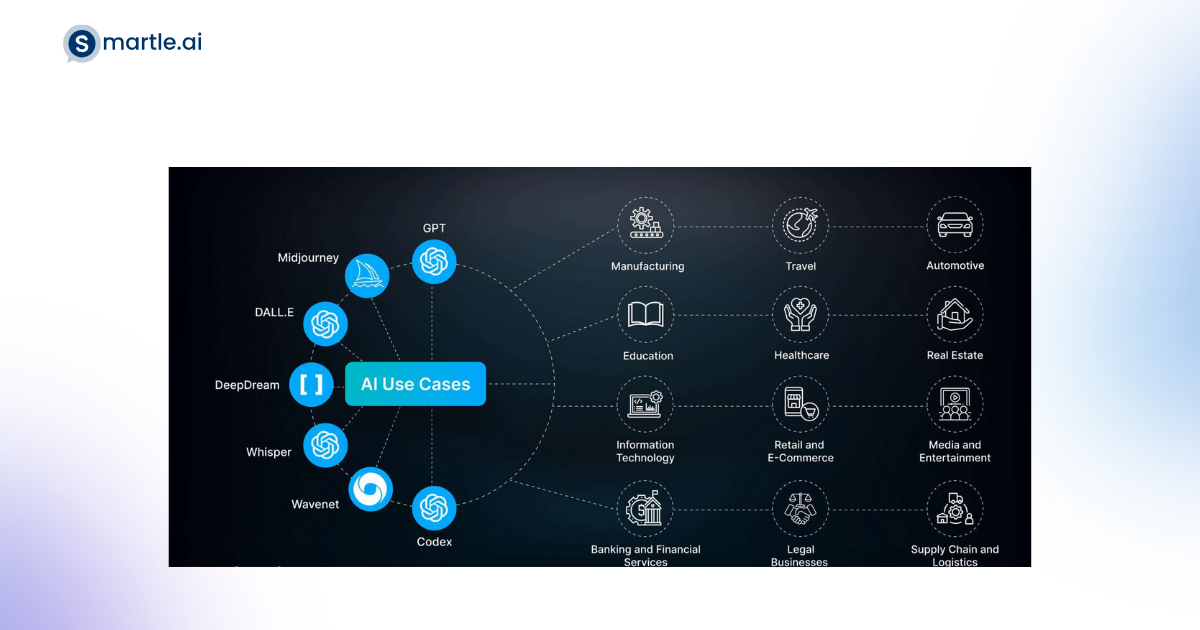
While tech giants pour billions into developing cutting-edge AI models, you don’t need a massive budget to take advantage of these innovations. By leveraging existing AI technology, businesses of all sizes can unlock powerful capabilities that drive growth and profitability. Here’s how you can tap into the power of billion-dollar AI models without breaking the bank:
- Piggyback on Industry-Leading AI: Instead of building AI systems from scratch, utilize pre-trained models like GPT-3 or GPT-4 through API integrations. This approach allows you to leverage the advanced capabilities of these models for tasks such as customer service, content generation, and data analysis. By using these APIs, you can implement sophisticated AI solutions that enhance customer interactions and streamline operations, all without the upfront costs associated with training a new model.
- Harness Retrieval-Augmented Generation (RAG): RAG combines the power of AI-generated content with real-time information retrieval, ensuring your AI always has the most up-to-date and relevant information. By using RAG, you can tailor responses to include the latest product details, promotions, and customer inquiries. This is particularly valuable for businesses that need to manage a dynamic inventory or provide precise, context-aware customer support.
- Use Case Example: An e-commerce platform using RAG can instantly pull product information from its database, providing accurate answers to customer queries about stock availability, product features, and delivery times, thereby improving customer satisfaction and boosting sales.
- Fine-Tune for Specific Applications: Fine-tuning allows you to customize pre-trained AI models to suit specific industry needs without the costs of developing an AI system from scratch. By training these models on domain-specific data, such as customer service transcripts or industry-specific terminology, businesses can create highly effective, specialized AI tools that enhance customer engagement and streamline business processes.
- Use Case Example: A healthcare provider could fine-tune a language model to better understand medical terminology and patient concerns, providing accurate information and support in healthcare settings.
- Adopt Open-Source AI Models: Open-source models, such as Meta’s LLaMA 2, offer a cost-effective way to access advanced AI technology. These models can be fine-tuned and adapted to fit specific business needs, enabling companies to harness state-of-the-art AI without expensive licensing fees.
- Use Case Example: An online retailer might use an open-source model to develop a personalized recommendation engine, analyzing customer behavior and preferences to suggest products that align with individual tastes, thereby increasing sales and customer loyalty.
- Smarter Data Strategies: Instead of focusing solely on the size of the dataset, prioritize data quality and relevance. Techniques like active learning, which focuses training on the most informative data points, can optimize model performance without the need for massive amounts of data. This approach not only reduces costs but also increases the efficiency and effectiveness of AI deployments.
- Use Case Example: A company might use smart data strategies to train a sentiment analysis tool that focuses on high-engagement customer reviews and feedback, gaining valuable insights to improve products and services.
By implementing these strategies, businesses can unlock the powerful capabilities of billion-dollar AI models, driving growth, enhancing customer experiences, and optimizing operations—all without the need for a massive R&D budget.
At Smartle.ai, we specialize in leveraging these advanced AI models to empower e-commerce owners. Our solutions provide real-time, personalized assistance that transforms casual visitors into loyal, high-value customers. With AI-driven recommendations and community commerce features, Smartle AI helps you maximize profits and create a thriving, engaged customer base. By making smart AI investments, you can harness the power of billion-dollar innovations and set your store apart from the competition.
The Million (Billion?) Dollar Question
As we watch tech giants and startups alike pour fortunes into the AI money pit, we’ve got to wonder: Are we on the brink of a revolutionary breakthrough, or are we watching the world’s most expensive digital bonfire?
What do you think? Is AI worth these astronomical costs, or are we due for a reality check? Drop your thoughts in the comments – who knows, your idea might just save the next AI company a cool billion or two!
Remember, the next time you’re chatting with an AI, you’re basically talking about something that costs more than some countries’ GDPs. Now that’s what I call an expensive conversation starter! 💸🤖